What is Downtime?
Downtime is a term used in manufacturing to describe the amount of time that an asset is not producing a product. This can be due to planned or unplanned downtime. Planned downtime occurs when a manufacturer shuts down certain equipment for routine maintenance and repairs, such as replacing worn-out parts or performing routine calibration checks. Unplanned downtime occurs when something goes wrong with the equipment and it needs to be fixed immediately.
Downtime is an important part of running a manufacturing facility because it can lead to lost revenue and increased expenses. For example, if one piece of equipment breaks down and needs immediate repair, your operations will be disrupted until the issue is resolved. This can slow down production or even stop it altogether until repairs are made.
Why is Downtime Analysis Important?
Downtime analysis is crucial for several reasons:
- Identify Root Causes: Downtime analysis helps to identify the underlying reasons for equipment failures and production stoppages. By understanding the root causes of downtime events, manufacturers can address issues at their source, rather than just treating the symptoms. This proactive approach allows for more effective problem-solving and prevents recurring downtime incidents.
- Improve Efficiency: Analyzing downtime data enables manufacturers to identify inefficiencies in their production processes. By pinpointing bottlenecks, equipment failures, and other sources of downtime, companies can implement targeted improvements to streamline operations, optimize workflow, and enhance overall efficiency. This leads to increased productivity and reduced operational costs.
- Optimize Maintenance Strategies: Downtime analysis provides valuable insights into equipment performance and maintenance needs. By analyzing patterns of downtime events, manufacturers can identify opportunities for preventive maintenance, predictive maintenance, or condition-based maintenance. Implementing proactive maintenance strategies based on data-driven insights helps to minimize unplanned downtime, extend equipment lifespan, and reduce maintenance costs.
- Enhance Decision-Making: Downtime analysis provides decision-makers with actionable information to support strategic planning and resource allocation. By understanding the impact of downtime on production schedules, customer orders, and revenue generation, managers can make informed decisions about resource allocation, production planning, equipment investments, and staffing levels. This ensures that resources are allocated effectively to maximize productivity and profitability.
- Drive Continuous Improvement: Downtime analysis fosters a culture of continuous improvement within an organization. By regularly analyzing downtime data and performance metrics, manufacturers can identify areas for improvement and track the effectiveness of implemented solutions over time. This iterative process of analysis, action, and evaluation allows companies to continuously optimize their processes, enhance performance, and stay competitive in a rapidly changing market.
Overall, downtime analysis is essential for maximizing operational efficiency, minimizing production disruptions, and driving continuous improvement across all aspects of manufacturing operations.
How do manufacturers perform downtime analysis today?
Manufacturers perform downtime analysis through a combination of manual data collection, automated monitoring systems, and sophisticated analytics tools. Here's an overview of the typical process:
- Data Collection: Manufacturers gather data on equipment downtime using various methods, including manual recording, automated sensors, and machine monitoring systems. Operators and maintenance personnel may manually record downtime events in logbooks or digital forms, documenting details such as the date, time, duration, reason for downtime, and actions taken to resolve the issue. Automated sensors and monitoring systems installed on equipment continuously collect data on machine status, performance metrics, and downtime events in real-time
- Downtime Classification: Once downtime data is collected, manufacturers classify downtime events into different categories based on the root cause or reason for the interruption. Common categories include equipment failures, maintenance activities, changeovers, material shortages, operator errors, and other unplanned events. By categorizing downtime events, manufacturers can identify patterns, trends, and recurring issues that affect production efficiency.
- Analysis and Visualization: Manufacturers use advanced analytics tools and software platforms to analyze downtime data and identify underlying patterns, trends, and root causes. These tools enable manufacturers to visualize downtime metrics through interactive dashboards, reports, and charts, providing insights into equipment performance, downtime frequency, duration, and impact on production output. Analytical techniques such as Pareto analysis, trend analysis, and root cause analysis help manufacturers identify the most significant contributors to downtime and prioritize improvement efforts accordingly.
- Root Cause Analysis: To address downtime effectively, manufacturers conduct root cause analysis to identify the underlying reasons or factors that contribute to equipment failures and production stoppages. Root cause analysis techniques such as Fishbone diagrams (Ishikawa), 5 Whys, Fault Tree Analysis (FTA), and Failure Mode and Effects Analysis (FMEA) help manufacturers systematically identify and address the root causes of downtime events. By understanding the root causes, manufacturers can implement targeted corrective actions to prevent recurring downtime incidents and improve overall equipment reliability.
- Continuous Improvement: Downtime analysis is an ongoing process that drives continuous improvement in manufacturing operations. Manufacturers regularly review downtime data, analyze performance metrics, and monitor key indicators to identify opportunities for optimization and enhancement. By implementing preventive maintenance strategies, predictive analytics, process improvements, and employee training programs, manufacturers strive to minimize downtime, maximize productivity, and enhance overall operational efficiency.
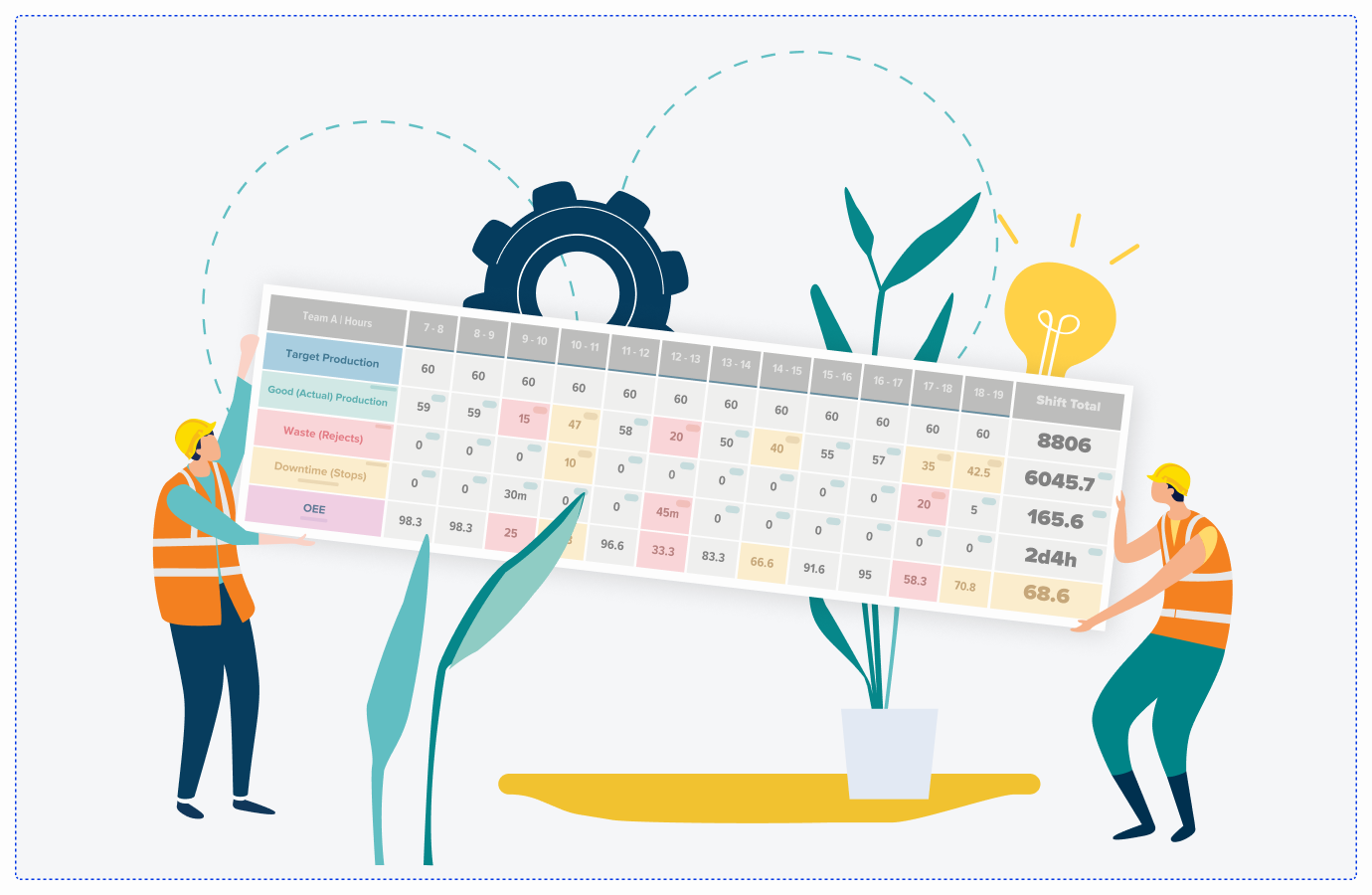
Plan Downtime with Digital Production Board
Some benefits of using ML and AI for downtime analysis
- Reduce unplanned downtime.
- Improve equipment effectiveness.
- Improve production efficiency.
- Improve quality, and thus increase profitability for your business as well as the customer experience (which will lead to repeat customers).
- Reduce cost of operations by reducing the need for manual labor, which can be very expensive in today's market.
The role of Machine Learning and Artificial Intelligence in downtime analysis
Machine Learning (ML) and Artificial Intelligence (AI) play a pivotal role in downtime analysis by leveraging advanced algorithms and predictive models to extract actionable insights from vast amounts of data. These technologies enable manufacturers to move beyond traditional approaches to downtime analysis and achieve more proactive and predictive maintenance strategies.
ML algorithms can analyze historical downtime data, equipment sensor readings, and other relevant variables to identify patterns, trends, and anomalies indicative of impending equipment failures or performance degradation. By continuously learning from new data inputs, ML models can predict potential downtime events with greater accuracy and anticipate maintenance needs before they occur. This proactive approach helps manufacturers minimize unplanned downtime, optimize maintenance schedules, and improve overall equipment reliability.
Furthermore, AI-powered analytics platforms can automate the detection, classification, and root cause analysis of downtime events, significantly reducing the time and effort required for manual data processing and analysis. AI algorithms can process large datasets in real-time, identify correlations between different factors contributing to downtime, and provide actionable insights to maintenance teams. By harnessing the power of AI-driven analytics, manufacturers can gain deeper visibility into their production processes, uncover hidden patterns or inefficiencies, and make data-driven decisions to optimize equipment performance and mitigate downtime risks.
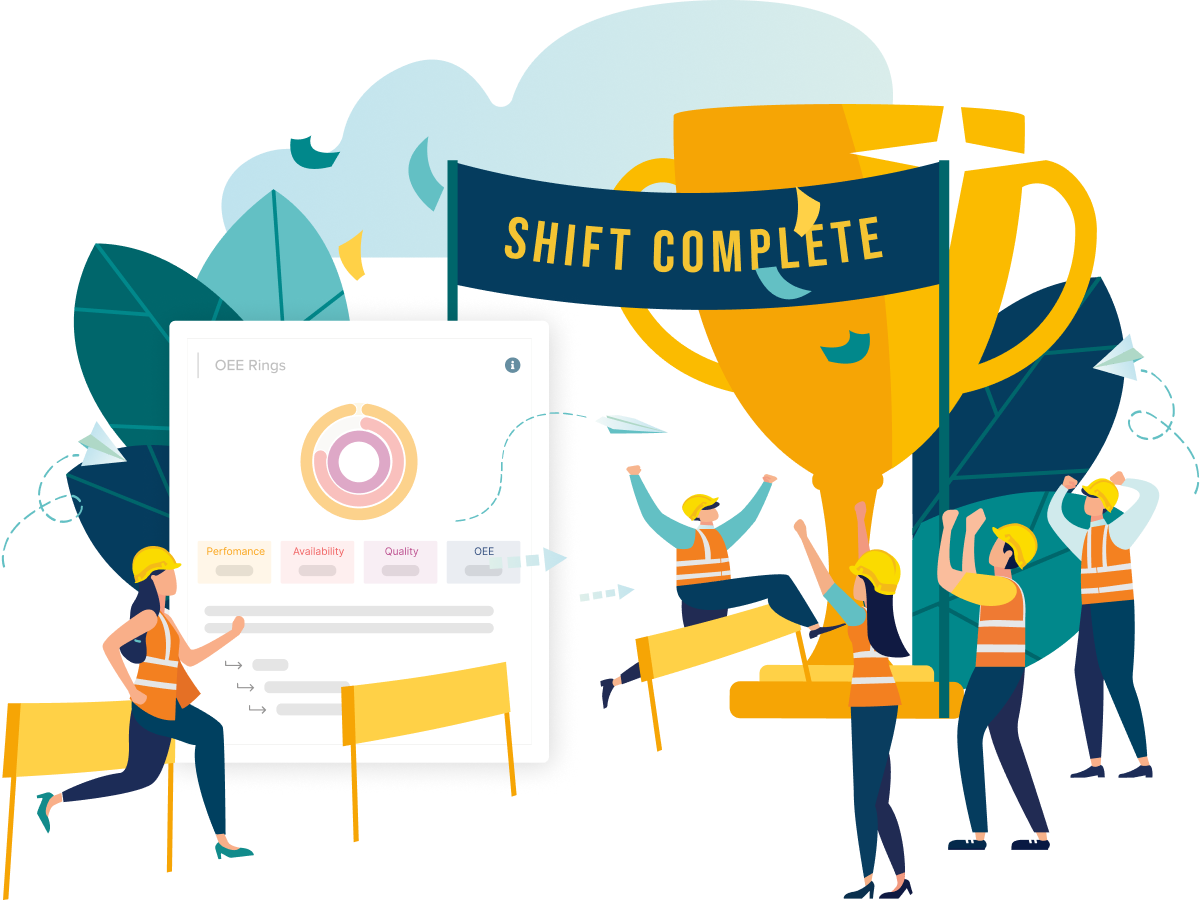
Shift complete success without Downtime
Analyzing Downtime Patterns and Root Causes
Analyzing downtime patterns and identifying root causes is a crucial aspect of downtime management and optimization. By delving deeper into the data collected through downtime analysis, manufacturers can uncover underlying trends, recurring issues, and the primary factors contributing to unplanned equipment downtime.
various criteria such as equipment type, production line, shift timing, and downtime duration. This classification allows manufacturers to identify common trends or patterns across different production areas and prioritize their efforts to address the most critical issues first. For example, they may discover that certain equipment failures tend to occur more frequently during specific shifts or operational conditions, providing insights into potential causes or contributing factors.
Additionally, conducting root cause analysis enables manufacturers to pinpoint the underlying reasons behind downtime events and implement targeted corrective actions to prevent recurrence. Root cause analysis involves investigating the sequence of events leading up to a downtime incident, examining equipment performance data, maintenance records, and operator feedback, and identifying the fundamental factors that triggered the downtime event. By addressing the root causes rather than just the symptoms, manufacturers can implement more effective solutions to minimize future downtime and improve overall equipment reliability.
Furthermore, advanced analytics techniques such as machine learning and predictive modeling can enhance the accuracy and efficiency of downtime pattern analysis and root cause identification. These technologies can analyze large volumes of historical downtime data, identify complex relationships between different variables, and uncover insights that may not be apparent through traditional analysis methods alone. By leveraging advanced analytics capabilities, manufacturers can gain a deeper understanding of their production processes, optimize equipment performance, and proactively mitigate downtime risks to ensure uninterrupted operations.
Conclusion
We hope that this article has given you a better idea of how machine learning and artificial intelligence can help manufacturers predict equipment failures, schedule maintenance, and reduce unplanned downtime. With the right tools and processes in place, it's possible to maximize uptime and increase productivity while minimizing costs associated with downtime analysis.